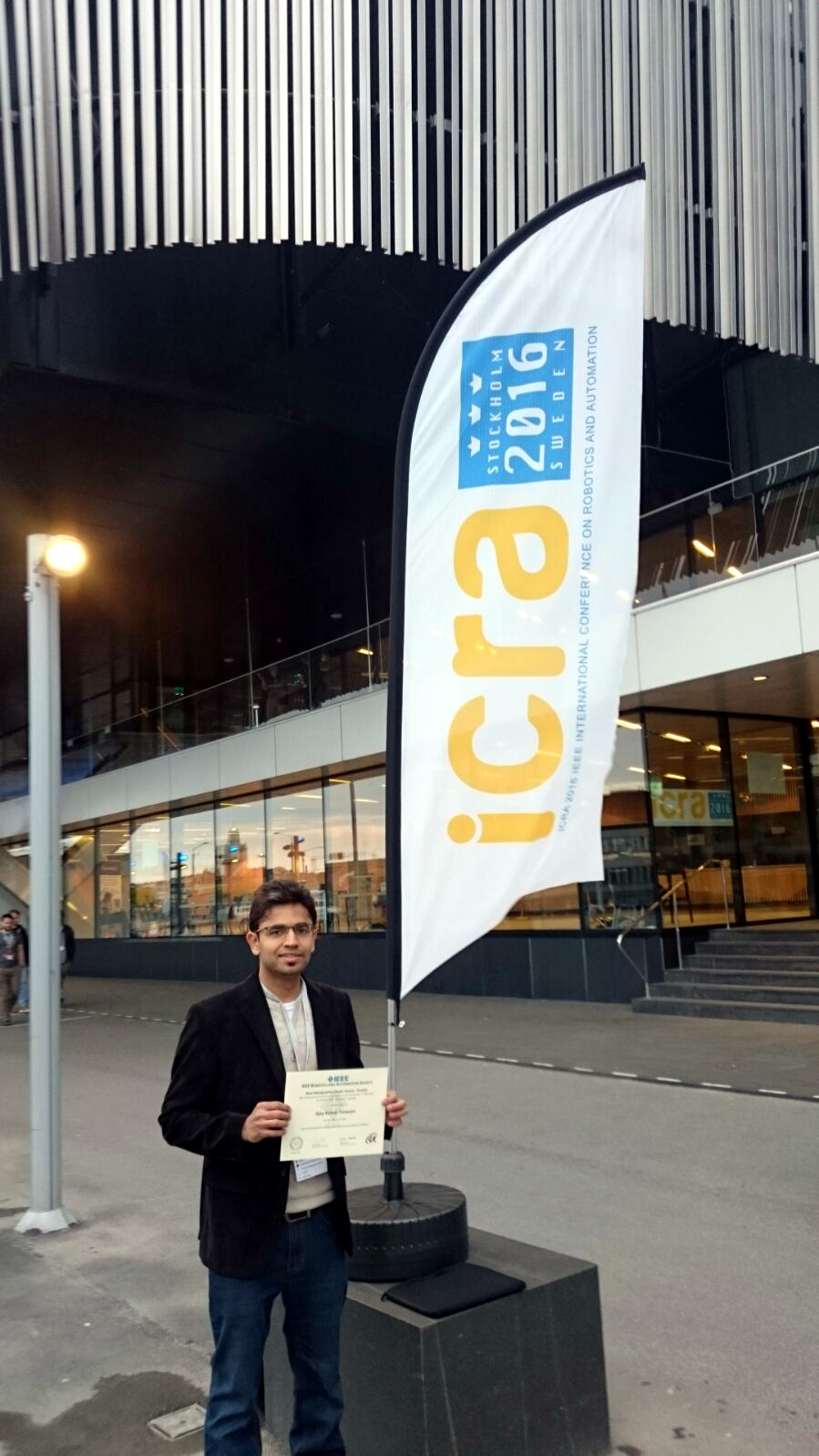
DexROV partner Idiap Research Institute has had their paper selected among award finalists at the IEEE International Conference on Robotics and Automation in Stockholm this month. The paper focused on Idiap’s research for the DexROV project, and Ajay Tanwani was officially recognised for the paper at the conference.
Titled “Learning Robot Manipulation Tasks with Task-Parameterized Semi-Tied Hidden Semi-Markov Model”, the paper was authored by Ajay Tanwani and Sylvain Calinon. Ajay is doing his PhD at Idiap/Swiss Federal Institute of Technology (EPFL). His recent work focuses on developing efficient and scalable generative models for skill acquisition in teleoperated robots. Together with Sylvain, they work with the Baxter robot to develop novel solutions to the unique DexROV challenge.
The IEEE International Conference on Robotics and Automation (ICRA’2016 ) was held in Stockholm, Sweden from 16 to 21 May. This year a record 2,357 submissions were registered, with 34.7% selected for the conference. The Idiap paper was selected as a finalist in the ‘Best Manipulation Paper Award’ category. Papers were judged on technical merit, originality, potential impact on the field, clarity of writing, and quality of presentation.
The paper describes a framework for a robotic learning model that converts movement from a human demonstration into autonomous robot manipulation tasks. The learning model exploits the coordinated movements in the environment (spatial) and in the timing (temporal) to share the parameters of the model in a “semi-tied manner”. In simple terms, the model groups movements together in segments that can be cleverly activated to generalise better over many more situations. The model is able to adapt itself according to changing manipulation scenarios to remotely adjust the movement of a robot while a teleoperator performs a task. The autonomous capabilities of the model fill any gaps in the movement caused by disconnections between the robot and the teleoperator.
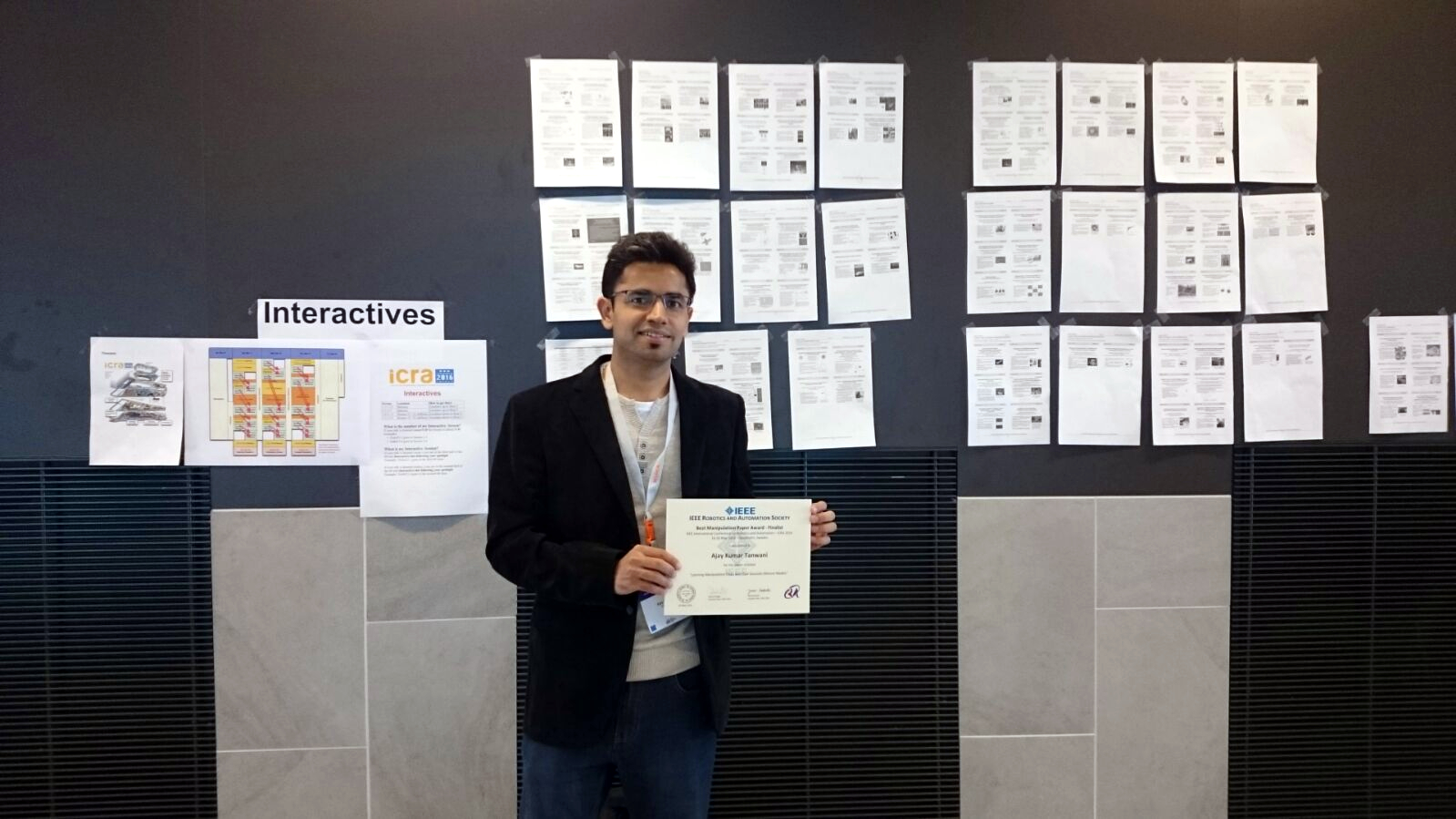
Using this model, the Baxter robot was taught to open a valve and pick-and-place an object while avoiding an obstacle after only four demonstrations of each task. The experiments with Baxter showed that the approach being used by Idiap is easily generalised with very compact coding and can adapt well to previously unexperienced situations.
The paper describes the DexROV scenario, where semi-autonomous teleoperations are crucial. DexROV will learn a generative model of movement from the demonstrations provided by the teleoperator in the on-shore control centre. The Idiap robotic automation model learns to recognise the movements of the teleoperator so that it can reproduce a similar motion for remote manipulation. DexROV will be able to undertake tasks in a semi-autonomous manner so that the teleoperator can work with the communication latencies that are a typical obstacle when using satellite communication.